Random graph models for real-world networks
Department Away Day `24
Joost Jorritsma



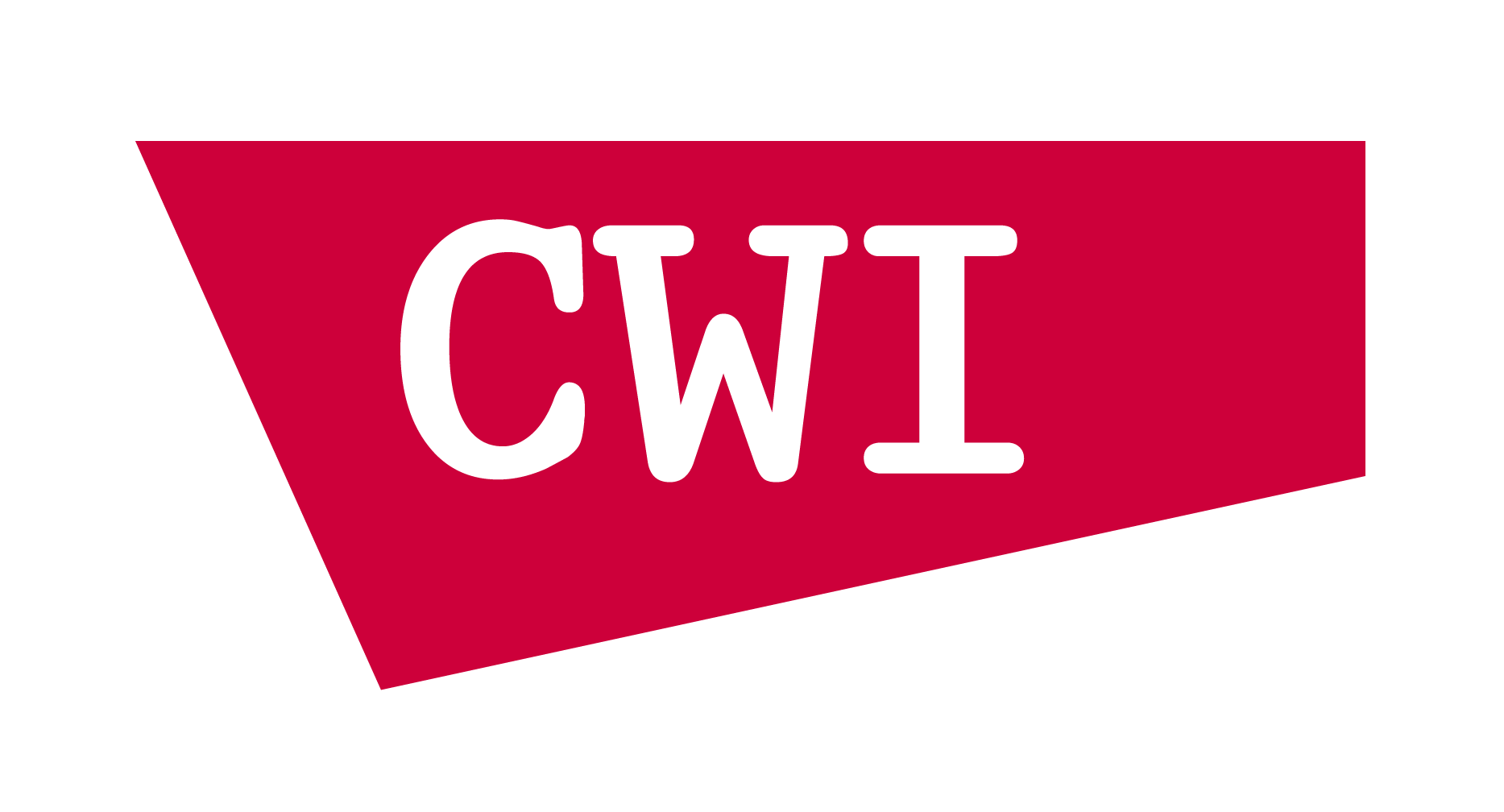


Math,
Music,
Biking






Understanding network models


Probability, Combinatorics
& Physics, Epidemiology, ...


Information diffusion in random graphs

Distances


Component sizes
Intervention strategies


FINAL SIZE
Randomized optimization algorithms
Optimization under chance constraints
Sampling algorithms for explainable AI
Inhomogeneous degrees, triangles, and small world












Inhomogeneous degrees, triangles, and small world












Inhomogeneous degrees, triangles, and small world












Inhomogeneous degrees, triangles, and small world
















Vertex set
- Spatial locations
- Vertex weights
Edge more likely if
- Spatially nearby,
- High weight
Spatial random graphs
Percolation: Reed-Frost epidemic






Four parameters: new and classical models
New phenomena
- Delocalized connected components
- Double-exponential neighbourhood growth
- Large outbreaks more likely than small outbreaks
Challenges
- Geometry:
- Short/Long edges
- Presence of hubs
New direction: Scaling limits of trees and random graphs
- Only vertex weights, no location
- Edge lengths scale with graph size

Figure by Igor Kortchemski
Setup
- Include asymmetry in edge probabilities
- Uncountable and unbounded weights
Goal
References
- The Continuum Random Tree; David Aldous; Annals of Probability (1991).
-
Limits of multiplicative inhomogeneous random graphs and Lévy trees: limit theorems;
Nicolas Broutin, Thomas Duquesne, Minmin Wang; Probability Theory & Related Fields (2021). -
Cluster-size decay in supercritical long-range percolation;
With Júlia Komjáthy, Dieter Mitsche; Electronic Journal of Probability (2024). -
Cluster-size decay in supercritical kernel-based spatial random graphs;
With Júlia Komjáthy, Dieter Mitsche; Min. revision in Annals of Probability, arXiv: 2303.00712. -
Large deviations of the giant in supercritical kernel-based spatial random graphs;
With Júlia Komjáthy, Dieter Mitsche; Preprint arXiv: 2404.02984. -
Surface order large deviations for Ising, Potts and percolation models;
Agoston Pisztora; Probability Theory and Related Fields (2024).
Department Away Day `24
Joost Jorritsma
Random graph models for real-world networks
Department Away Day `24
Joost Jorritsma
Internet: a growing network of routers and servers
~1969: 2 connected sites
Time
~1989: 0.5 million users
~2023: billions of devices
- [Faloutsos, Faloutsos & Faloutsos, '99]:
-
Short average distance:
Quick spread of information
-
Short average distance:

~1999: 248 million users
Distance evolution in a growing network
1999
\(\mathrm{dist}_{\color{red}{'99}}(u_{'99}, v_{'99}) = 4\)
2005
\(\mathrm{dist}_{{\color{red}'05}}(u_{'99}, v_{'99}) = 3\)
2024
\(\mathrm{dist}_{{\color{red}'24}}(u_{'99}, v_{'99}) = 2\)
21 possible networks
Attachment rule:
Prefer connecting to high-degree vertices, \(\tau\): tail of power-law degree distribution
2005
\(\phantom{\mathrm{dist}_{{\color{red}'05}}(u_{'99}, v_{'99}) = 3}\)
Distance evolution
Distance evolution: hydrodynamic limit
Theorem [J., Komjáthy, Annals of Applied Probability '22]. Assume \(\tau<3\).
Let \(t'=T_t(a):=t\exp\big(\log^a(t)\big)\) for \(a\in[0,1]\), then
$$ \sup_{a\in[0,1]} \left| \frac{\mathrm{dist}_{T_t(a)}(U_t, V_t)}{\log\log(t)} - (1-a)\frac{4}{|\log(\tau-2)|}\right|\overset{\mathbb{P}}\longrightarrow 0.$$
Theorem [J., Komjáthy, Annals of Applied Probability '22]. Assume \(\tau<3\).
Let \(\phantom{t'=T_t(a):=t\exp\big(\log^a(t)\big)}\) for \(\phantom{a\in[0,1]}\), then
$$ \phantom{\sup_{a\in[0,1]} \left| \frac{\mathrm{dist}_{T_t(a)}(U_t, V_t)}{\log\log(t)} - (1-a)\frac{4}{|\log(\tau-2)|}\right|\overset{\mathbb{P}}{\longrightarrow} 0.}$$
Theorem [J., Komjáthy, Annals of Applied Probability '22]. Assume \(\tau<3\).
Let \(t'=T_t(a):=t\exp\big(\log^a(t)\big)\) for \(a\in[0,1]\), then
$$ \phantom{\sup_{a\in[0,1]}} \left| \frac{\mathrm{dist}_{T_t(a)}(U_t, V_t)}{\log\log(t)} \phantom{- (1-a)\frac{4}{|\log(\tau-2)|}}\right|\phantom{\overset{\mathbb{P}}\longrightarrow 0.}$$
-
Dynamics in PAMs.
-
Generalization with edge weights: random transmission times
Novelties
-
Fast spreading among influentials;
Theorem [J., Komjáthy, Annals of Applied Probability '22]. Assume \(\tau<3\).
Let \(t'=T_t(a):=t\exp\big(\log^a(t)\big)\) for \(a\in[0,1]\), then
$$ \sup_{a\in[0,1]} \left| \frac{\mathrm{dist}_{T_t(a)}(U_t, V_t)}{\log\log(t)} - (1-a)\frac{4}{|\log(\tau-2)|}\right|\phantom{\overset{\mathbb{P}}\longrightarrow 0.}$$
Theorem [J., Komjáthy, Annals of Applied Probability '22]. Assume \(\tau<3\).
Let \(t'=T_t(a):=t\exp\big(\log^a(t)\big)\) for \(a\in[0,1]\), then
$$ \phantom{\sup_{a\in[0,1]}} \left| \frac{\mathrm{dist}_{T_t(a)}(U_t, V_t)}{\log\log(t)} - (1-a)\frac{4}{|\log(\tau-2)|}\right|\phantom{\overset{\mathbb{P}}\longrightarrow 0.}$$
Information diffusion in random graphs

Distances


Component sizes
Intervention strategies


FINAL SIZE
Real networks contain many triangles!

Do real networks look like this?

Large deviations (rare events) of cluster sizes
Kernel-based spatial random graphs


Only four parameters
Vertex set
- Spatial locations
- Vertex weights
Edge more likely if
- Spatially nearby,
- High weight




Hyperbolic random graph
Scale-free percolation
Long-range percolation
Bond percolation on \(\mathbb{Z}^d\)

Theorem (\(\mathbb{Z}^d\)-like graphs)
[Lebowitz & Schonmann '88; Gandolfi '89; Grimmett, Marstrand '90;
Kesten, Zhang '90; Alexander, Chayes, Chayes, Newman '90; Pisztora '96;
Cerf '97; Contreras, Martineau, Tassion '2024; ...]
Lower tail:
Surface tension drives too small cluster
Upper tail:
Large clusters are very unlikely
Figure by Tobias Muller




Hyperbolic random graph
Scale-free percolation
Long-range percolation
Bond percolation on \(\mathbb{Z}^d\)

Theorem (\(\mathbb{Z}^d\)-like graphs)
[Lebowitz & Schonmann '88; Gandolfi '89; Grimmett, Marstrand '90;
Kesten, Zhang '90; Alexander, Chayes, Chayes, Newman '90; Pisztora '96;
Cerf '97; Contreras, Martineau, Tassion '2024; ...]
Lower tail:
Surface tension drives too small cluster
Upper tail:
Large clusters are very unlikely
Question:
Long edges and high-degree vertices,
do they matter?
Theorem [J., Komjáthy, Mitsche, '24+]
We find explicit \(\zeta\in[1/2,1)\), \(\theta\in(0,1)\) s.t.
-
Lower tail: small final size
If enough long edges or no hubs

Novelties
-
Reversed discrepancy: large outbreak likelier than small.
-
Long edges can beat surface tension: any ;
-
governs second-largest cluster, and cluster of 0
-
Techniques: probability, combinatorics, optimization.
What is the influence of long edges and high-degree vertices?
-
Upper tail: large final size
If hubs are present, we find rate funtion \(I(\varepsilon)\):
What is the influence of long edges and high-degree vertices?
Information diffusion in random graphs

Distances


Component sizes
Intervention strategies


FINAL SIZE
Research plan: Large deviations in percolation and random graphs
Ongoing
Near future
Opportunities Leiden
- Large deviations of the giant in inhomogeneous random graphs
Bert Zwart (CWI) - Tall or small trees
Serte Donderwinkel (Groningen)
- Applying for CIRM Fellowship
Luisa Andreis (Milan), - MSc Students (Oxford)
- Dalia Terhesu

Research plan: Random walks on random graphs
Ongoing
Opportunities Leiden

- Random friend of a friend tree
Sofiya Burova (PhD student Barcelona),
Dieter Mitsche (Santiago de Chile) - Cover time of long-range percolation Carlos (MSc student Lima), D. Mitsche
- (Evolution of) the mixing time of preferential attachment models (PAM)
- Generating PAMs via random walks
Rajat Hazra & PhD student
Building a network: Attend and organize workshops, seminars
Near future (invitations)
- One-world probability seminar
- Probability meets Combinatorics (IST, Vienna)
- Long-range phenomena in Percolation (Köln)
- Mathematical Foundations of Network Models and their Applications (Chennai)
Opportunities Leiden: Lorentz Center
Organisational experience:
RandNET Workshop (with Serte Donderwinkel)
- 10 days, 80 participants
- Mini-courses, Open problem sessions (4+ papers)
- Budget: €55k
- Attending prof:
"You set the gold standard for running such an event"
Department Away Day
By joostjor
Department Away Day
- 223